Model Risk Management: A Comprehensive Overview
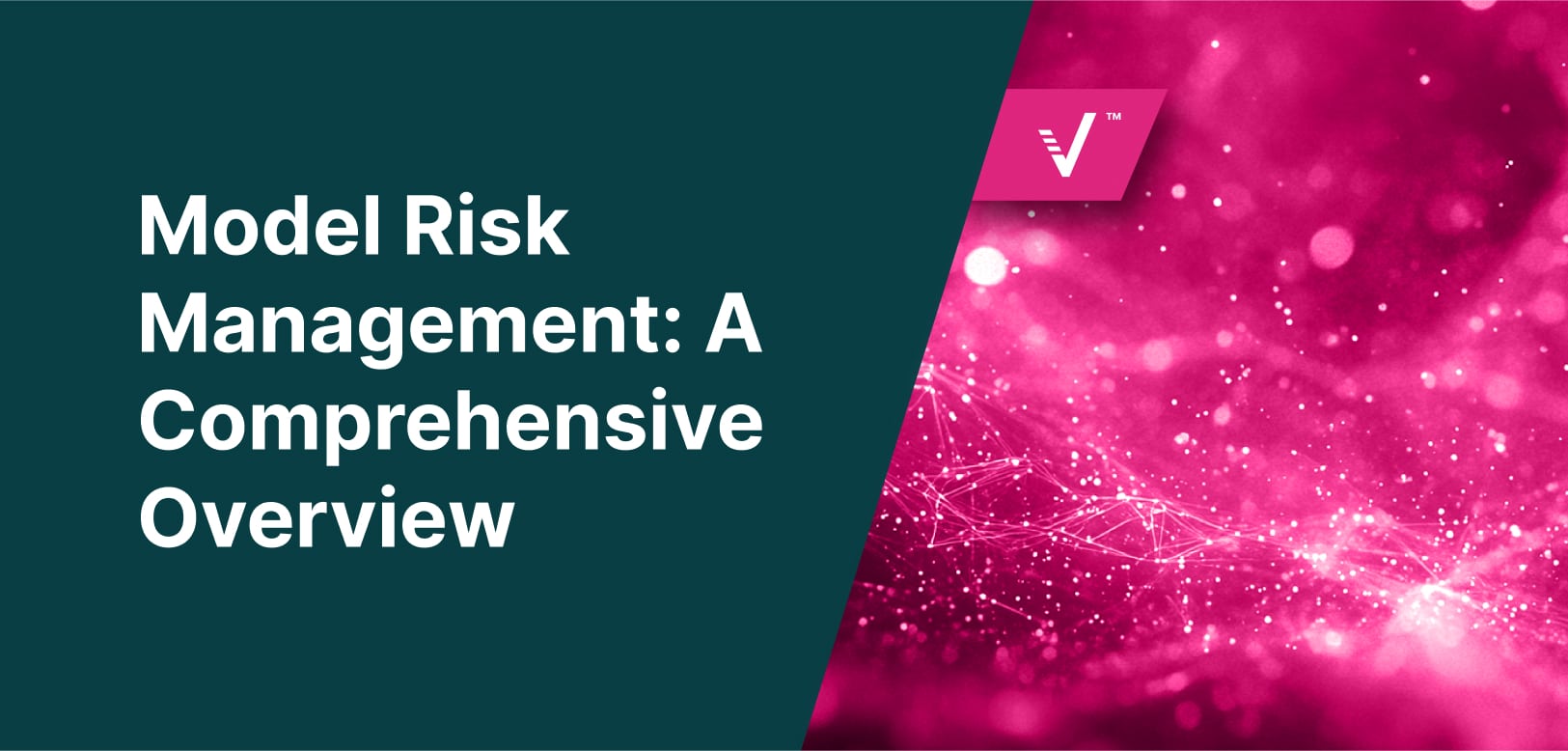
Model risk management as a discipline emerged in the wake of the 2008 financial crisis, when financial models used by Wall Street banks and investors wreaked havoc on the economy.
As much as the term “model” has always applied to simple representations of complex systems, like a plastic model of a fighter jet or a cardboard model of a multi-million dollar building, the term is creaking under the weight of artificial intelligence.
In the 2020s, the emergence of large language models into the mainstream has created a whole new category for what constitutes a model. While quantitative models prior to AI would never be described as “simple,” AI models based on neural networks—such as the brain—are phenomenally complicated.
If businesses, financial institutions, and governments were already struggling to deal with the complexity and risk of more established models for credit, liquidity, pricing, and the likes, they are now scrambling to quantify, manage, and regulate the risk from these advanced AI models.
In this article, we’ll lay the foundations for understanding risk from various models and how organizations should approach model risk management (MRM). We’ll also cover technology tools and trends that will affect the trajectory of model risk management frameworks in the marketplace.
The Model Risk Management Origin Story
Basic mathematical modeling is as old as the disciplines of architecture and engineering. It relies on the ability to project a future reality based on known quantities and the interactions of those quantities.
Complex mathematical models in finance go back at least to the 1990s, but their origins may reach as far back as the 1970s.
Quantitative models became commercially accessible alongside desktop and mainframe computers, although the most advanced models required the use of supercomputers.
Scrutiny of models and their effect on the broader society hit a fever pitch with the financial crisis of 2008. Investors and regulators overlooked the catastrophic risks posed by derivatives markets of mortgage-backed securities (MBS), credit-default swaps (CDS), and collateralized debt obligations (CDOs). The fallout nearly triggered a systemic collapse of the global banking system.
The 2008 crisis highlighted the two dominant causes of risk from mathematical models:
- Fundamental errors in the structure of the model: Some models were based on a speculation that house prices would continue to rise, and failed to use sufficient historical data on prices.
- Misapplication of the model’s output: While in theory the derivatives markets spread risk around and helped create security, in reality, they just obscured the problems and then magnified them.
One of the aspects to take away from this failure is that the validity of the models was established on the credibility of the institutions that employed them, rather than independent scrutiny. After the financial crisis, regulators across the globe realized that models would need strict oversight to prevent similar crashes.
As a consequence, the U.S. Federal Reserve Bank (the Fed) issued SR 11-7 in 2011, which provided initial guidance on model risk management (MRM) for U.S. banks.
Although the Bank for International Settlements (BIS) issued additional guidance on risk management in the same month that Lehman Brothers failed, it didn’t provide a detailed framework for MRM.
Basel II provided some guidance about the use of models and associated risks. Then, in 2010, Basel III laid out more detailed expectations about how institutions should manage model risk.
But it wasn’t until 2017 that the Prudential Regulation Authority (PRA) of the Bank of England and the European Central Bank issued their formal guidance on model risk management.
The Federal Reserve and Office of the Comptroller of the Currency (OCC) has led the regulation of MRM at financial institutions. Their definition of a model is succinct and coherent:
“The term model refers to a quantitative method, system, or approach that applies statistical, economic, financial, or mathematical theories, techniques, and assumptions to process input data into quantitative estimates.”
Although at the time it was published the guidance didn’t mention AI or machine learning (ML), these techniques have come under the regulatory umbrella of SR 11-7.
Despite the advanced complexity of quantitative models used for investing and banking, they pale in comparison to the size, scope, and methodology of AI/ML models. The pool of experts who understand the structure and development of AI/ML models is small compared to the ranks of quantitative analysts (“quants”) who manage other financial models.
This gap in comprehension has driven the need for software—such as ValidMind—that can automate the rigors of MRM across conventional models, and rapidly advancing AI models.
What Is Model Risk Management?
The British statistician George E. P. Box is renowned for saying, “all models are wrong, but some are useful.” While a model is an attempt to create a realistic future scenario with enough accuracy to help make business decisions, they’re always incomplete.
In fact, models are particularly good at illustrating probable outcomes, rather than predicting precise outcomes.
Because they can’t possibly incorporate all the complex interactions of the real world, risk is inherent to models when they are used as a guide for decisions. If the guidance is wrong, the practical outcome is likely to reflect that through monetary loss—as well as other costs.
The three types of model risk that regulators hope to mitigate are:
- Flaws in the model’s design
- Inaccurate data
- Misapplication of the model’s output
SR 11-7 stipulates that institutions need to establish formal processes for model development, validation, monitoring, governance, and testing of internal models.
Model risk management encompasses the various processes and procedures that companies and institutions have used to comply with SR 11-7. With the 2021 release of OCC’s Model Risk Management Handbook, institutions now have a well-defined map for what U.S. regulators expect to see when they audit MRM practices.
MRM Lifecycle: The Five Stages
MRM is primarily focused on the early, formative stages of a model’s lifecycle, but it includes the later refining stages as well.
Stage 1: Development
It’s not uncommon for software developers to document their process as they build a platform or application. However, documentation is a requirement for institutions or third-party vendors developing models. This creates the transparency that auditors need to evaluate the model’s structural integrity.
Stage 2: Validation
SR 11-7 requires that model developers employ independent validation (it can be internal or external, but the validation role must be separate from development) of the model’s performance in different scenarios. Validation is somewhat analogous to a cybersecurity penetration test, as you look for vulnerabilities in the model.
Stage 3: Monitoring
Once a model is deployed to a live environment, SR 11-7 requires continuous monitoring, to watch for model drift and outputs that fall outside of the accepted nominal range.
Stage 4: Governance
It’s not enough to establish policies and procedures for MRM; SR 11-7 requires proof that an institution is adhering to those policies and they’re in line with the latest regulatory guidance. Institutions must clearly separate the various team functions to ensure proper accountability.
Stage 5: Testing
The majority of testing happens during the development stage of a model, but it may be used additionally to spot-check the model for issues. Platforms such as ValidMind allow institutions to employ pre-built, compliant tests as well as custom tests.
Some institutions may feel that MRM regulations add an unnecessary burden to the model development process. However, the principles are sound and, if followed, lower the risk of significant losses and reputational harm.
One of the best ways to comply with MRM regulations without overburdening your team is to find a partner who can assist with tedious and mundane tasks, such as documentation or providing independent validation.
Why Is Model Risk Management Important?
The systemic implications of unmanaged model risk were proven in 2008 when a few bad (and/or naive) actors threw the global financial system into chaos.
One of the most valuable roles that regulators can play is to create and enforce standards that are unlikely to arise voluntarily within the industry. MRM is a prime example. The public can’t be expected to grasp the finer details of financial models, and the market can’t be relied on as a corrective force (unless we accept catastrophic corrections as a normal occurrence).
Model risk is akin to herd immunity. You need each individual actor to demonstrate good health in order to prevent contagion. Once you have widespread immunity, the herd is protected from errant cases of disease.
By mandating MRM to institutions and third-party vendors, regulators are seeking to create individual accountability that translates into systemic resilience and stability.
The benefits of MRM may seem self-evident, especially because the penalties are now a double-tap: once from market losses and again from regulators.
Even though MRM regulation introduces new friction to the model development and deployment processes, it functions as a protective measure for the institution or vendor. Robust models deliver better results to clients and the bottom line. Weak models corrode brand reputations and lead to other punishments.
Key Components of Model Risk Management
MRM intends to identify risks early on, remediate those risks when possible, and ensure long-term alignment with an institution’s goals and applicable regulations.
MRM is the most effective and efficient when it’s integrated with the lifecycle of the model and automated wherever possible.
Model Development
Model development is notoriously difficult in the best of circumstances, and the requirement to document the development process can feel like it steals from more important work.
All models benefit from continuous improvement, but you need a strong foundation if the model is going to perform well over the long term.
Development is the first stage where risk gets introduced, in the forms of failure to adequately define the business objective, faulty structural assumptions, or even poor training data. Proper documentation allows model developers to review the critical steps, identify issues, and remediate those issues quickly.
That’s why it’s so valuable to integrate a platform like ValidMind into your development process: so that it can provide the documentation that auditors want, while your team focuses on building the best model possible.
Model Testing
Testing is a vital part of the development process. The reason that the MRM discipline formalizes it is to ensure that tests are robust, consistent, and transparent.
Rigorous testing helps to prove a model’s alignment with business objectives and an organization’s risk appetite, while stress testing is specifically for proving a model’s resilience against outlying data that it may not have been designed to handle.
Your testing regimen is an excellent opportunity to bring in an independent party for fresh perspective and accountability.
Model Validation
Validation is akin to a fact-checker review of a newspaper article prior to publishing. According to SR 11-7, the validation role needs to be independent of the development team, albeit with full access to the development data and documentation.
This allows the validators to review the model’s assumptions and methodologies to see whether it performs as claimed, and aligns with corporate and regulatory standards.
Validation should include:
- Backtesting: Running the model on historical data to see if it accurately reproduces the intended outputs.
- Sensitivity testing: Tweaking parameters and inputs to see how robust the model is compared to standard deviation.
- Benchmarking: Comparing the performance of a model with comparable models to evaluate how it differs and why.
The purpose of independent validation is to unearth biases and deficiencies in the development process that are difficult for the development team to self-edit. It ensures real-world performance rather than theoretical or ideal scenario performance.
Model Monitoring
Although it’s easy to confuse with testing, monitoring happens after deployment. It’s a record of the model’s performance using real-world data. Monitoring is the best way to flag any drift in the model (or hallucinations in the case of large language models and generative AI).
Model monitoring includes period reviews and stress-testing, as well as calibration or updates where necessary.
When institutions fail to monitor the output of a model (i.e., “set it and forget it”), they inject unnecessary risk into their operation. Software automation can provide continuous monitoring and help maintain the human review cadence required to stay in compliance.
Model Governance
Governance is simply the formal accountability to establish policies and procedures and follow them.
While regulators may stipulate some rules in great detail, they usually provide high-level guidance and require institutions to practice what they preach (or document in formal policies and procedures).
SR 11-7 and other regulations do lay out proper governance structures, with the explicit acknowledgment that institutions are working with human and capital resource constraints. Healthy governance includes establishing model risk committees, model ownership roles, and compliance with internal controls.
Putting such governance in place helps to create sufficient independence in the MRM organization without creating silos or eliminating checks and balances.
The Challenges of Modern Model Risk Management
Most institutions recognize the need for MRM, but they face external and internal challenges when establishing or improving their MRM processes and organization. Here are five challenges to pay attention to.
1. Legacy Systems and Infrastructure
Many financial institutions rely on manual processes and systems for everyday operations. Given the fast-paced development of models and the shifting regulatory environment, this poses a huge disadvantage.
MRM is unsustainable and unscalable without modern technology to automate and document what’s happening.
2. Data Quality and Availability
Much as everyone likes to think of data as made up of “rights” and “wrongs,” data integrity and availability pose a massive challenge for any financial institution. Without clear processes for standardization and hygiene, it’s nearly impossible to gather enough high-quality data for proper model training.
This contributes to one of the primary model risks: poor data.
3. High Costs and Resource Constraints
According to the St. Louis Fed, large banks devote 2.9% of their noninterest expense to compliance, while small banks devote three times that much (8.7%).
The added expense of building out an MRM organization on a budget stretched thin by existing regulations is a grim prospect for many institutions.
Some institutions are accustomed to asking staff to wear multiple hats. For MRM compliance, this may not be realistic. SR 11-7 lays out the need for distinct roles that do not overlap. In many cases, bringing in a third party can solve two problems at once: keeping headcount low and expenses within budget.
4. Regulatory Complexity and Compliance
No financial institution wants to deal with adverse action from a regulator. The penalties rack up quickly, and the rules are often open to interpretation, making violations feel unfair at times.
When compliance feels like a sinkhole for money and time, MRM becomes one more “must-do” on an endless to-do list. Not to mention that most of the models at work fall outside the expertise of the employees tasked with performing MRM. This phenomenon is likely to intensify as the use of advanced AI/ML models becomes more widespread.
5. Model Complexity
Most quantitative models were already the purview of quantitative analysts, and far outside the comprehension of the average bank employee. AI models push that dynamic even further.
Sometimes, AI models are called “black box models” because they’re so difficult to understand, validate, and explain. Manual validation methods aren’t sufficient or scalable. AI models require AI validation or equivalently sophisticated software that is built to address AI model complexity.
Very few institutions are equipped to tackle these challenges in-house, let alone try to build an internal team with the right expertise.
The Role of Technology in Model Risk Management
The first step toward addressing the demands of MRM is to assess the challenges.
Then, you can begin evaluating the tools and solutions that will help you succeed. Technology plays an integral role in model development and deployment.
It can also help free up your team to focus on the highest-value work—the less time your developers spend documenting their work, the more time they can spend refining the model. Here are six ways technology drives modern MRM.
1. Automation of Core MRM Processes
Technology provides the ideal tools and framework to conduct MRM and automate tasks such as documentation, validation, and monitoring.
Now that robust MRM platforms and software have been introduced to the marketplace, it’s way more efficient to adopt those tools than to develop your own from scratch.
2. Centralized Model Inventory
One of the requirements of SR 11-7 is for institutions and companies to maintain a complete and up-to-date inventory of all the models they use. Using a centralized digital platform makes it much easier to track the relevant information about each model and where it’s deployed.
A digital repository can also serve as the central hub for your MRM efforts, providing a single source of truth for everyone, including auditors.
3. Enhanced Monitoring and Reporting
Continuous monitoring and reporting on model performance is a massive and tedious undertaking. AI-driven platforms such as ValidMind are designed to watch your models, flag issues, and generate reports automatically.
Regular reporting is a vital aspect of MRM for internal stakeholders, as well as external parties such as auditors and partners, but it isn’t sustainable to manually generate all the necessary documents.
5. Integration of AI and ML
It may sound surprising at first, but the only technology sophisticated enough to properly test, validate, and monitor AI is another AI model that’s built for that exact purpose.
ValidMind’s platform helps you create transparency and accountability for all your models, especially the “black box” models that require expert analysis.
6. Regulatory Compliance
Financial regulations may not be easily comprehensible, but they’re fundamentally a system of rules.
ValidMind’s platform is built to enforce those rules throughout the MRM process. As new regulations get released, ValidMind’s team incorporates them into the platform so you don’t have to worry about it.
Your whole team needs to respect the regulations, but they don’t have to memorize them if you use technology to guide the MRM process.
Regulatory Landscape and Model Risk Management
Globalism and technology have created a deeply connected network of financial systems.
A financial or economic crisis in any of the world’s largest countries is likely to have spillover effects.
While most countries have dedicated regulatory entities for banks and financial services, some regulatory bodies are consortiums, such as the Bank for International Settlements (BIS) and the European Central Bank (ECB).
SR 11-7 (U.S. Federal Reserve)
As the central bank for the largest economy in the world, the Fed provides both financial services (primarily to other U.S. banks but also to the U.S. government) and regulatory guidance.
In the aftermath of the 2008 financial crisis, the Fed issued SR 11-7 in 2011 to establish a comprehensive MRM regime for financial institutions. SR 11-7 dictates the need for independent validation, regular monitoring, and proper governance throughout a model’s lifecycle.
Basel II/III (Global)
The BIS, a consortium of central banks, including the Fed, issued the guidance of Basel III in 2010. It expanded on earlier MRM guidance from Basel II for models and created specific requirements for assessing the risk posed by models, including credit risk, market risk, and operational risk.
Basel III also requires banks to maintain adequate capital reserves based on model outputs, which increases the importance of robust and compliant models.
European Banking Authority (EBA) Guidelines
For the Eurozone, the EBA also provides guidance on MRM, which echoes SR 11-7 in many respects. The EBA’s rules focus on the importance of transparency, traceability, and the documentation of model changes, validation, and risk.
Additional Regulations
Other regulatory bodies have issued additional guidance for their jurisdictions, as well as to address emergent fields such as AI:
- The United Kingdom – Prudential Regulation Authority (PRA): SS1/23 Model Risk Principles.
- Canada – Office of the Superintendent of Financial Institutions Canada (OSFI): E-23 Enterprise-Wide Model Risk Management for Deposit-Taking Institutions.
- The European Union – The European Commission: The EU AI Act.
The intent of these regulations is to protect against catastrophic, irreversible, and systemic damage inflicted by financial and AI models.
Penalties for non-compliance with these regulations are designed to function as a proverbial “stick” for financial institutions and corporations. The upside, or “carrot,” is that healthy MRM can lead to significantly better performance and brand reputation.
Platforms such as ValidMind can help organizations avoid the regulatory stick as much as possible and maximize the carrot.
Model Risk Management Trends To Pay Attention To
While the public conversation around models is focused on the novelty of AI, the reality is that advanced model development is entering many industries with far-reaching consequences.
Here are some trends that will affect MRM in direct and indirect ways:
1. AI and Machine Learning Integration
As you may have noticed, since ChatGPT opened for use in 2022, the speed of AI development has been moving at a blistering pace. Many institutions were already looking for ways to deploy AI/ML in credit underwriting and other types of complex financial decisions.
While large language models (LLMs) will continue to attract publicity for their analogous human capabilities, the discipline of AI/ML is set to transform much of how we live and work.
2. Explainability and Transparency (XAI)
An outpouring of the rapid advancement of AI models is the cry for explainable AI (XAI). Due to the vast and intricate structure of AI models, it’s extremely difficult to provide a straightforward answer for how the model arrived at an output.
Although some AI applications (such as filtering spam emails) don’t really need to explain their output, an AI model that provides credit decisions on loan applications is legally required to demonstrate that it isn’t violating laws such as the Equal Credit Opportunity Act (ECOA).
Although the precise definition of XAI isn’t laid out in the regulatory guidance, the concept will continue to inform how regulators engage with MRM.
3. Increased Regulatory Scrutiny and New Guidelines
The latest regulations for MRM won’t be the final word. You won’t find a crystal ball (or AI model) that can predict what future regulations will look like, but many of the priorities for MRM are clear enough:
- Transparency
- Traceability
- Robustness
- Fairness
- Accountability
- Explicit risk assessments
- Strong governance
Individual regulations will vary, but if your institution is pursuing MRM in good faith, with sufficient technology to support your efforts, the next batch of rules will feel like a hiccup instead of a hurricane.
4. Automation and Real-Time Monitoring
Models scale fast. People don’t.
As more and more models deploy, financial institutions and their vendors need to adopt scalable MRM processes, especially for documentation, validation, and monitoring. ValidMind’s platform is designed to build out and maintain an MRM process that works for your organization.
5. Generative AI in Financial Models
We mentioned the hallucinogenic tendency of LLMs and generative AI (GenAI) earlier in this piece.
LLMs such as ChatGPT are probabilistic and sound matter-of-fact, but are often unable to distinguish fact from fiction. This presents a unique set of risks that quantitative financial models don’t have at the same level.
Many institutions are exploring ways to use GenAI to enhance the customer experience online and in the branch. Using an MRM platform such as ValidMind can help you manage GenAI models and address their unique risks.
Model Risk Management Shouldn’t Feel Overwhelming
Yes, models, and MRM, are complicated matters.
That’s why no one should attempt an overnight transformation of existing (or non-existing) MRM practices. Building a mature MRM team takes time, education, and the right technology.
Your first step should be to evaluate your current MRM environment:
- Are you currently using financial models?
- What MRM policies or procedures do you have in place?
- How prepared is your team for an audit?
Don’t get stuck in the minutiae, but don’t ignore painful truths—regulators certainly won’t.
Lastly, you should open conversations with vendors in the MRM space. Even if you’re planning to build out a dedicated MRM team, you still need to equip them with the right tools for the job.
ValidMind has built a model risk management platform that takes the most burdensome aspects of model risk compliance and automates them.
We don’t build models, but we can help you make the most of every model you deploy.
Contact us to discover how ValidMind makes MRM a breeze.