How Model Risk Management (MRM) Teams Can Comply with SR 11-7
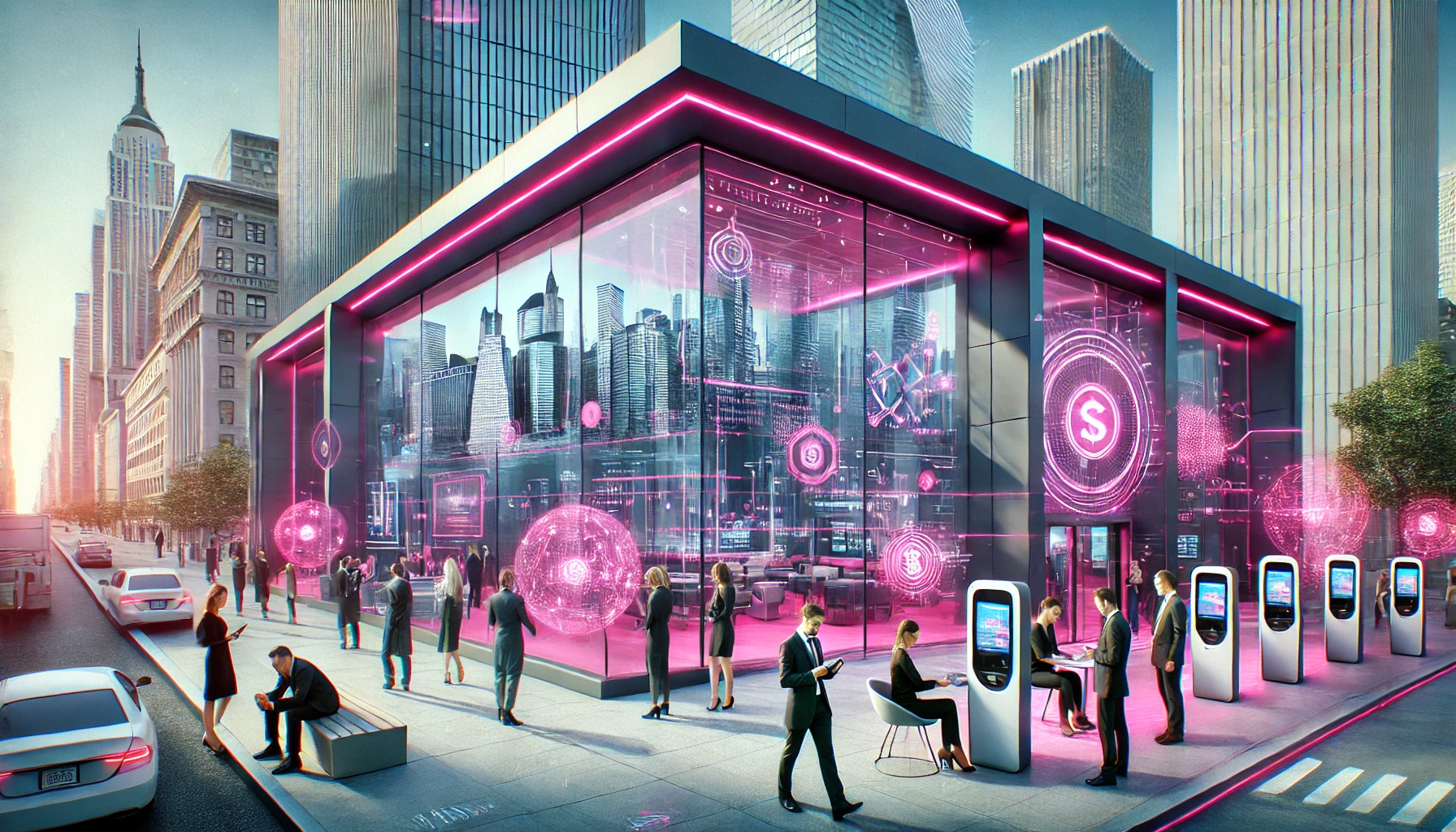
For more than a decade, regulation SR 11-7 — Supervisory Guidance on Model Risk Management — has been keeping MRM teams busy, requiring them to adhere to a set of guidelines created to mitigate model risk. The US Federal Reserve created these guidelines in an attempt to outline the key concepts of model risk management as it pertains to all banking organizations within the Federal Reserve.
As emerging technologies continue to gain prominence (including artificial intelligence (AI), generative AI (GenAI), large language models (LLMs), and machine learning (ML)), MRM teams’ ability to comply with SR 11-7 becomes increasingly complex.
SR 11-7 Compliance for Model Risk Management Teams
There is always a fluctuating level of inherent risk and uncertainty involved when using AI and statistical models within banking operations. While different financial institutions may have varying levels of appetite for risk, there’s rarely if ever, a zero-risk financial model. Risks tend to arise for two main reasons: foundational errors resulting in faulty outputs or incorrect model application.
Both of these scenarios can harm a bank’s reputation profoundly, and a poorly tested, improperly validated model can potentially cost a bank millions of dollars.
The SR 11-7 framework provides a structure that allows for strong model oversight, which helps banks minimize risk. Today’s modern, innovative banks are finding success in complying with SR 11-7 by creating a robust culture of sound MRM practices that include best practices for Model Validation, Model Documentation, Model Governance, and Monitoring.
Model Validation
MRM teams must maintain organization within the model development process and ensure that it aligns with the policies of the user and banking organization. Model validation plays a crucial role here. It involves paying close attention to how the model is integrated into the system by thorough documentation and review, and continuously monitoring the model to verify it is working as intended and that its results align with your goals.
Flexible risk assessment is also important as the specifics of a validation depend on how often the model is used and how complex it and the bank is. SR 11-7 stresses the importance of understanding how the model acts and how to minimize and control model risk.
Through proper model validation, potential risks are easily identified, documented, and mitigated, ensuring the model is robust and dependable.
AI and Statistical Model Governance
A strong governance framework is necessary to ensure that the model complies with its intended purpose. Teams must consistently identify, document, and validate the risks that come with third-party models and assess whether the models meet internal goals as outlined in the SR 11-7.
To help, implement a governing body within the MRM operation, such as a board or senior management, and hold them accountable for MRM activities to ease the validation process. This includes establishing policies for MRM teams through organized responsibility and oversight.
This governance structure would guarantee that all aspects of the model (i.e. its development, usage, etc.) would be systematically controlled and cooperate with bank requirements and other regulatory factors. This reduces issues related to the model and promotes compliance with SR 11-7.
ValidThoughts Webinar Replay | Navigating MRM Challenges: Strategies for Compliance
Model Documentation
Comprehensive documentation is another key factor in a successful, robust model risk management program. The SR 11-7 requires exhaustive documentation of the model validation process, current development, and usage to maintain operation and clarity for all parties involved.
Proper documentation provides the team with a direct understanding of current activity, a concise audit trail, and a detailed review of the models. Even people unfamiliar with the model should be able to understand how it operates, its capabilities and limitations, and the reasons for its use.
This also helps in evaluating the model’s compliance with the banks’ operational standards, taking into account its impact on auditors, regulators, and other stakeholders involved in the banking process.
Monitoring AI and Statistical Models
To keep up with market conditions or changes in regulatory requirements, constant monitoring is needed. MRM teams regularly review the model’s performance to ensure that the model is meeting its intended use. Teams use model inventories that documents which models are currently in use, under development, or retired.
Continuous monitoring helps detect any mishap or failures within the model, providing the team with time to intervene and prevent any negative impacts on the bank.
Conclusion
Adhering to the SR 11-7 guidelines is important for Model Risk Management (MRM) teams to maintain proper validation, governance, detailed documentation, and monitoring of models used in banking operations.
Want to learn how innovative financial institutions are adhering to SR 11-7? Speak with one of our MRM experts today by filling out our contact form.