More on Uncertainty: Responding to a16z’s Generative AI Challenge for Financial Services
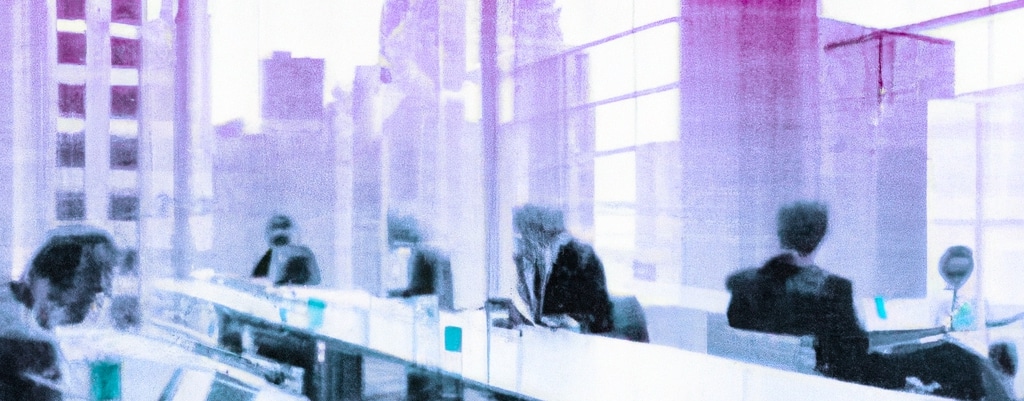
The team at a16z, which includes Angela Strange, Anish Acharya, Sumeet Singh, Alex Rampell, Marc Andrusko, Joe Schmidt, David Haber, and Seema Amble, recently published their post Financial Services Will Embrace Generative AI Faster Than You Think. In this post, they outline what could be “the largest transformation the financial services market has seen in decades.“
The combination of access to a massive amount of historical financial data, both personal and corporate, combined with the ability to train Large Language Models (LLMs), as Bloomberg has done with BloombergGPT, could mean tremendous business opportunities. That said, as the a16z team explains in their post, one of the key challenges for generative AI models, such as LLMs, is the correctness or appropriateness of the generated content, whether textual responses or predictions:
“Given the impact the answer to a financial question can have on individuals, companies, and society, these new AI models need to be as accurate as possible. They can’t hallucinate, or make up, wrong but confident-sounding answers to critical questions about one’s taxes or financial health, and they need to be far more accurate than the approximate answers for popular culture queries or generic high school essays. To start, there will often be a human in the loop as a final verification for an AI-generated answer.”
Output accuracy is particularly challenging when considering the uncertainty of a model. Model uncertainty is the lack of confidence or ambiguity associated with the predictions made by a machine learning model. It reflects the model’s level of uncertainty or doubt in its predictions. As explained in ValidMind’s recent blog post, model uncertainty can arise from various factors, including the limits of the training data, the complexity of the task, or the model’s inability to handle out-of-distribution examples.
The challenge with generative AI stems from the current prevalence of models with low accuracy and low uncertainty. In such cases, the model may be overconfident in its incorrect predictions, failing to acknowledge its own limitations or mistakes, as users of Bing AI found out recently. The implications and potential consequences of this phenomenon in financial services is particularly significant. For instance, it could result in offering misguided financial advice to customers or potentially lead decision-making systems to make biased decisions.
By contrast, high accuracy models that exhibit low uncertainty produce highly appropriate predictions or responses that are reliable and can be trusted.
What’s next for financial services?
In a regulatory context, we must understand that the concept of accuracy is only meaningful when the actual value is accessible or can be determined. As discussed in our recent series of articles on uncertainty, it is also important to highlight that the technique employed to derive uncertainty from ML or AI models can significantly impact the explainability of model output.
To address these challenges, the financial services industry must exercise caution and consider appropriate safeguards when employing generative AI models. This effort includes:
- Rigorous documentation, validation, and testing procedures
- Integrating domain expertise in model training and evaluation
- Incorporating external data sources for verification
- Implementing human oversight and review processes to ensure the accuracy and reliability of the generated outputs
Regulatory frameworks and compliance standards specific to financial services can also play a crucial role in ensuring transparency and accountability using generative AI models.
For more details, you can check out our series on understanding model uncertainty: